Implementing AI Expectations vs Reality
- firstformydata
- Dec 17, 2023
- 7 min read
Updated: Dec 12, 2024
The Truth About the Effectiveness of AI: Bridging the Gap Between Hype and Reality in 2025
In 2025, AI is no longer a buzzword; it’s a strategic imperative. Yet, despite its transformative potential, 85% of AI initiatives and use case fail to deliver measurable results and business value. Why? Unrealistic expectations, unprepared infrastructure, and a lack of clear objectives often derail progress. This article goes beyond the hype, offering actionable insights to help your business bridge the gap between ambition and execution—transforming an AI implementation strategy from a lofty goal into a competitive advantage
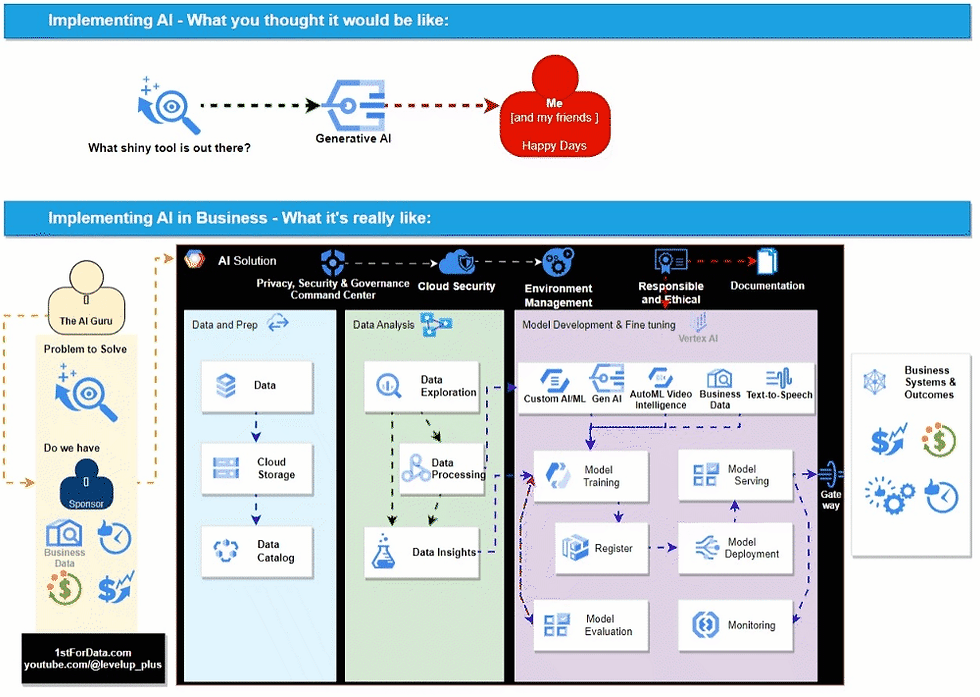
The promise of successful implementation of AI in business has sparked enthusiasm across industries, from insurance and finance to retail and healthcare. Businesses dream of the benefits of AI such as automated workflows, personalized customer experiences, and unprecedented efficiency. However, the journey from adopting responsible AI to achieving real value is anything but straightforward.
A staggering 85% of Artificial Intelligence projects fail to meet expectations, leaving many businesses frustrated and skeptical. But why does this happen? More importantly, how can businesses overcome these challenges and benefit from the impact of AI and its capabilities with their goals?
In this article, we’ll explore the gap between expectations and reality in AI implementation, uncover key factors for success, and share actionable strategies to bridge the divide. By the end, you’ll be armed with insights to make AI align with your business.
The AI Dream: A Game-Changer for Businesses
AI promises transformative outcomes, making it one of the most coveted technologies in modern business. Leaders envision a future where the use of AI effortlessly drives business operations:
Faster decision-making through predictive analytics.
Improved customer experiences via personalization and automation.
Cost savings from operational efficiency.
New revenue streams by uncovering untapped opportunities in data.
This vision is supported by a surge in AI investment. According to IDC's FutureScape Report, global spending on AI is expected to exceed $628 billion annually by 2028, fueled by its adoption across industries like insurance, banking, and retail. Businesses are focusing on AI-enhanced tools, such as predictive analytics and generative AI, to address complex challenges and stay competitive. To learn more, you can explore IDC’s findings
Yet, with great expectations comes great disappointment. Many companies soon discover that the path to AI implementation success is fraught with challenges, therefore choose the right AI and data strategy.

Where AI Expectations Meet with Reality
In 2025, AI will continue to be a transformative force in business, but implementing it is rarely as straightforward as companies hope. Many organizations begin their new journey anticipating seamless integration, only to encounter complex systems, steep learning curves, and unforeseen obstacles.
According to a Gartner report on AI failures, approximately 80% of projects fail to meet their goals due to issues such as unclear objectives, poor data quality, and a shortage of skilled talent. Recognizing and addressing these challenges is essential to bridging the gap between expectations and success.
Common AI Expectations
Plug-and-Play Solutions
Many businesses expect AI tools to work out of the box. However, the most effective AI systems require significant customization to meet specific organizational needs.
Instant ROI
AI is often viewed as a fast track to profitability, but the reality is that achieving returns requires substantial initial investment in data, infrastructure, and talent. Benefits frequently take years to materialize.
Minimal Human Involvement
AI is sometimes imagined as a hands-off solution, but it requires skilled professionals to train models, interpret results, and ensure ethical compliance.
The Harsh Realities
Lengthy Implementation Timelines
When you implement AI into your business it is rarely quick or straightforward. Developing robust data pipelines, training models, and integrating a robust AI solution into existing workflows often takes months—or even years. Unrealistic expectations about timelines are a leading cause of project delays or failures.
High Costs and Slow ROI
The steps for successful AI implementation demands significant upfront costs, including software, hardware, and specialized expertise. These investments, combined with ongoing maintenance, mean that realizing ROI is often a long-term endeavor.
Heavy Reliance on Expertise
AI success depends on access to skilled teams, including data scientists, machine learning engineers, and industry experts. However, the ongoing global shortage of new AI talent is a major hurdle for many organizations.
Bridging the Gap
To avoid falling into the 80% of failed AI integrations, organizations must adopt a pragmatic approach:
Define Clear Goals: Establish measurable objectives aligned with broader business strategies.
Invest in Data Readiness: Ensure high-quality, well-organized data is available for AI models.
Hire or Upskill Talent: Build teams with the expertise needed to manage AI integration effectively.
Set Realistic Timelines: Plan for long-term implementation and adapt as challenges arise.
By setting realistic expectations and investing in the right foundations, businesses can unlock AI's potential and achieve sustainable success.
Read more about the implementation process!
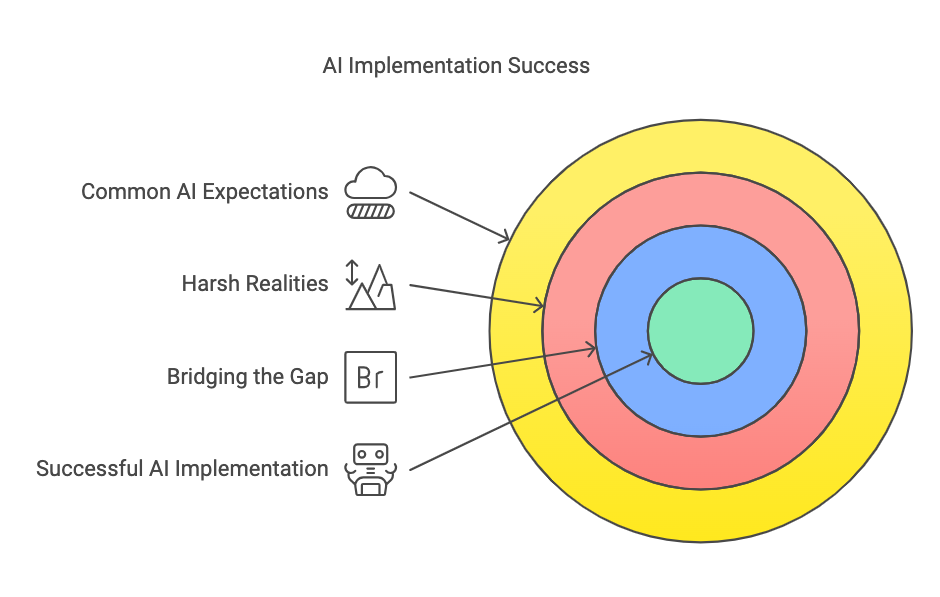
Why Do AI Projects Fail?
Identifying the root causes of AI and machine learning implementation failures is crucial for success. Below are the most common pitfalls:
1. Insufficient Data Readiness:
AI algorithms thrive on data, but most businesses lack clean, organized, and adequately labeled datasets. Without this foundation, even the most advanced algorithms can’t function effectively and align with your business objectives.
Example: A retail company trying to integrate AI for demand forecasting may struggle with fragmented sales data across regions, leading to inaccurate predictions and wasted resources.
2. Misalignment with Business Goals:
Implementing AI in business often fails because it's not aligned with clear, measurable KPIs. Investing in AI for the sake of “staying ahead” leads to unfocused projects that don’t deliver tangible value.
Example: An insurance firm implements and tests the effectiveness of training AI to automate underwriting without understanding the regulatory implications, resulting in delays and compliance issues. Its all about selecting the right ai model
3. Underestimating Technical Complexity:
AI solutions are rarely off-the-shelf products. Building, training, and deploying different AI systems require significant technical expertise, which many organizations lack.
4. Neglecting Ethical and Governance Concerns:
Ethical issues, such as biased algorithms or inadequate data privacy measures, can derail projects. Companies that don’t address these challenges risk losing customer trust and facing legal consequences.
Example: A facial recognition system trained on biased datasets may yield inaccurate results, leading to public backlash and regulatory scrutiny.
5. Overlooking Change Management:
AI implementation often requires cultural and operational shifts, yet many businesses fail to prepare their teams for these changes. Resistance to new processes can hinder adoption and impact outcomes.

Understanding where the challenges lie is the first step; now let’s dive deeper into how to successfully implement AI
Strategies for Successful AI Implementation
Despite the challenges, businesses can achieve AI success by taking a strategic, thoughtful approach.
1. Start with a Clear Vision
AI implementation in your business should begin with well-defined goals. Ask yourself:
What problem are we solving?
How will success be measured?
How does AI align with our broader business strategy?
Example: Instead of integrating AI across the board, an e-commerce company might focus on using AI for personalized product recommendations, targeting improved conversion rates as the key metric.
2. Invest in Data Readiness
Quality data is the lifeblood of AI. Organizations must invest in robust data management practices, including:
Cleaning and organizing existing datasets.
Building secure data pipelines.
Ensuring compliance with privacy regulations like GDPR or CCPA.
3. Upskill Your Workforce
AI isn’t just about technology—it’s about people and Ai model training. Businesses should invest in training employees to work alongside the right AI or hire professionals skilled in data science, machine learning, and AI governance.
4. Take an Incremental Approach
Large-scale AI transformations are risky. Start with small pilot projects to test viability, learn from challenges, and refine your approach before scaling up.
Example: A healthcare provider might first deploy AI for administrative tasks, such as appointment scheduling, before moving on to diagnostic support systems.
5. Focus on Ethics and Compliance
When you implement Artificial Intelligence in your business, addressing ethical concerns builds trust and ensures long-term success. Key considerations include:
Developing bias-detection frameworks.
Implementing robust privacy policies.
Ensuring transparency in how AI models make decisions.
6. Collaborate with Experts
Partnering with AI consultants like LevlUp and its top notch AI technologies, can fast-track your journey. From assessing needs to implementing solutions and training teams, their expert guidance ensures you’re business processes set up for success.
The Role of LevlUp in Bridging the Gap
At LevlUp, we specialize in guiding businesses through the complexities of AI implementation. Here’s how we help:
Needs Assessment: We work closely with clients to understand their goals, challenges, and industry-specific requirements.
Custom Solutions: Our team designs tailored AI solutions that align with business objectives while addressing compliance and governance needs.
Collaborative Execution: We collaborate with internal teams to implement AI systems in a phased, results-driven manner.
Empowering Teams: Through training and ongoing support, we ensure your workforce is equipped to manage and maintain AI systems.
Case in Point: AI in Insurance
Our expertise shines in industries like insurance, banking and enterprise where AI implementation can bring efficiencies in underwriting, claims processing, and fraud detection. By focusing on a tailored strategy, compliance and best practices, we’ve helped clients unlock the transformative potential of AI applications.
The Future of AI: Evolving Beyond the Challenges
While the challenges of implementation today are significant, the landscape is evolving rapidly. By 2025, advancements in technology and strategy are expected to address many of the current hurdles, making it more accessible and effective for businesses of all sizes. Here’s what the future holds:
Simplified AI Development: Tools like AutoML and no-code platforms are expected to streamline AI implementation, empowering non-technical teams to develop and deploy AI solutions without extensive coding expertise.
Enhanced AI Ethics and Compliance Frameworks: As regulations catch up with technology, more robust frameworks will emerge, helping businesses address concerns around bias, privacy, and governance with greater confidence.
Scalable AI Infrastructure: Innovations in cloud computing and edge AI will make systems more scalable and cost-effective, reducing barriers to entry for smaller businesses.
Integration with Emerging Technologies: AI will increasingly integrate with technologies like blockchain and IoT, creating opportunities for new applications and efficiencies across industries.
Upskilled Workforces: Organizations are investing in education, creating a future workforce equipped to collaborate effectively with machine learning tools.
By preparing for these advancements now, businesses can position themselves to leverage the full potential of AI as it continues to evolve.
Key Takeaways:
AI implementation is not plug-and-play: Success requires careful planning, robust data pipelines, and skilled teams.
Common pitfalls to avoid: Unrealistic expectations, lack of clear goals, and underestimating ethical challenges lead to most failures.
Long-term vision is essential: Align projects with measurable goals and set realistic timelines to achieve ROI.
Future advancements will simplify adoption: Tools like AutoML and better governance frameworks will lower barriers, making it more accessible and efficient.
Collaboration is key: Partnering with experts and investing in talent development can help businesses unlock AI’s full potential.
Conclusion
Adopting AI is not a quick fix—it’s a journey that demands careful planning, investment, and commitment. Business stakeholders must look beyond the allure of advanced technology and focus on best practices for AI::
Aligning with clear business goals.
Building robust data and infrastructure.
Addressing ethical concerns to build trust.
Developing skilled teams to manage it effectively.
By taking these steps, companies can bridge the gap between expectations and reality, creating long-lasting value and staying ahead in a competitive landscape.
Next Steps
Ready to transform your Artificial Intelligence strategy? Let’s talk - LevlUp can guide you every step of the way? Arrange a Readiness and Strategy Session now!
Comments